· Tutorials · 4 min read
Predicting Pandemic Peaks
In the early days of a pandemic, the world was plunged into uncertainty. Governments urgently needed to predict how the virus would spread and the impact of interventions like social distancing. To make these predictions, they turned to mathematicians and data analysts. But the question was, how could these experts provide reliable forecasts amid so many unknowns?
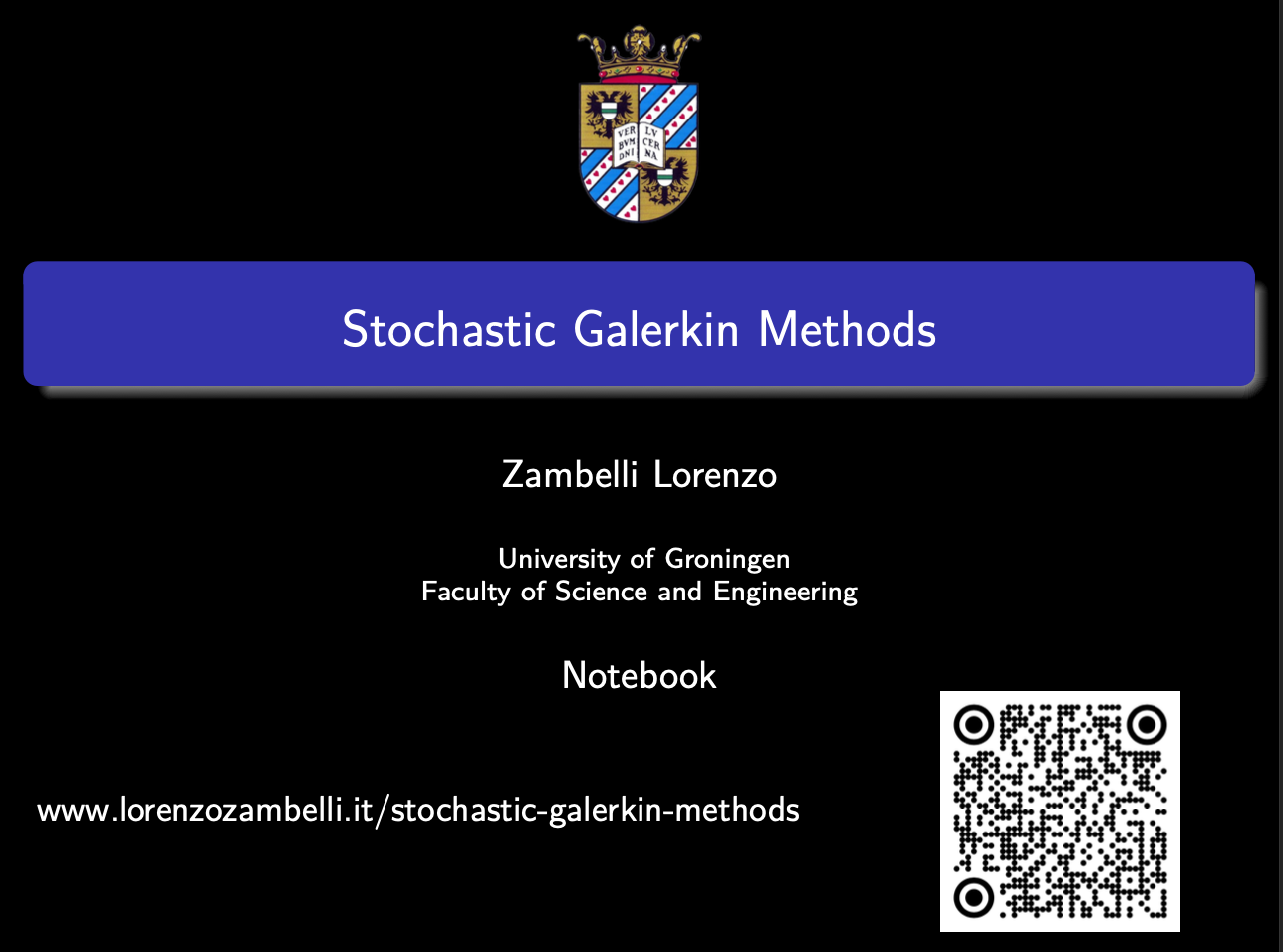
Mathematicians use models to simulate real-world phenomena. The equations one gets from a mode describing the spread of a virus are called Partial Differential Equations (PDEs), which involve the rates at which quantities like the number of infected individuals change over time and space. These models help in understanding and predicting the dynamics of an epidemic.
One common model used in epidemiology is the SIER model, which stands for Susceptible, Infected, Exposed, and Recovered. This model divides the population into four compartments:
- Susceptible (S): Individuals who can catch the disease.
- Exposed (E): Individuals who have been exposed to the disease but are not yet infectious.
- Infected (I): Individuals who are infectious.
- Recovered (R): Individuals who have recovered and are immune.
The SIER model can be described using PDEs that account for the rates at which individuals move from one compartment to another. For example, the rate at which susceptible individuals become exposed depends on the contact rate and the number of infectious individuals. This model becomes more complex when adding dimensions like the location of infected individuals or constraints such as social distancing and mask-wearing.
To solve these complex PDEs, mathematicians often use the Galerkin Method, first developed by the Russian mathematician Boris G. Galerkin. Imagine you have a big, intricate puzzle, but you decide to solve it by breaking it into smaller, simpler pieces. The Galerkin Method works similarly: it approximates the solution by breaking down the problem into simpler functions, usually polynomials.
The basic idea is to express the complex solution as a sum of these simpler functions and then adjust the coefficients to best fit the original problem. This approach makes the problem more manageable and easier to solve with computers.
The key to the Galerkin Method is orthogonal projection. Think of orthogonal projection as fitting a bumpy curve with a series of straight lines. The lines are positioned in such a way that they minimize the overall error (distance between the curves and the lines). In the Galerkin Method, this means projecting the complex problem onto a set of basis functions (the simpler functions) that are orthogonal, or perpendicular (they form a 90-degree angle), to each other. This ensures that the approximation spreads the error evenly and makes the solution as accurate as possible.
In simpler terms, mathematicians discovered a neat trick. They found that any function, no matter how complex, can be approximated by adding up a series of simpler, orthogonal polynomials. Imagine trying to draw a complicated curve by connecting straight lines. As you use more lines, your drawing gets closer to the actual curve. Similarly, adding more polynomials makes the approximation more accurate. This process of getting closer and closer is called convergence, and it means that the difference between the actual solution and our approximation becomes very small as we include more terms.
In real-world scenarios, especially in a pandemic, there is always uncertainty. Factors like varying transmission rates and differences in population behavior introduce randomness into the model. Stochastic Galerkin Methods (SGMs) extend the traditional Galerkin Method to handle these uncertainties effectively.
So far we have learned how to determine the spread of a virus, but how close will this result be to the true infection? A remarkable theorem was first noted by American mathematicians Cameron and Martin in their 1944 article “Transformations of Wiener Integrals under Translations”. The theorem says that in a special kind of mathematical space called a Hilbert space, you can use a series of orthogonal polynomials to get very close to any function. The more terms you add to this series, the closer you get. When we say it “converges in the sense,” we mean that if you measure the difference between the actual function and the series using a specific mathematical tool, this difference gets very small as you add more terms.
Conclusion
Stochastic Galerkin Methods offer a powerful and efficient way to quantify uncertainty in differential equations, making them invaluable for predicting phenomena like virus spread. By leveraging the orthogonality of polynomial chaos expansions, SGMs provide precise and computationally affordable solutions. As we continue to navigate an uncertain world, methods like SGMs will be at the forefront of robust and reliable computational tools.
For those interested in diving deeper, the technical and theoretical details discussed here can be explored further in accompanying Jupyter notebooks and a presentation.